Can the use of digital technology improve the cow milk productivity in large dairy herds? Evidence from China's Shandong Province
- 1College of Economics and Management, Nanjing Agricultural University, Nanjing, China
- 2School of Agriculture and Environment, College of Sciences, Massey University, Palmerston North, New Zealand
- 3College of Economics and Management, Huazhong Agricultural University, Wuhan, China
- 4School of Digital Media and Humanities, Hunan University of Technology and Business, Changsha, China
Introduction: Improving milk productivity is essential for ensuring sustainable food production. However, the increasing difficulty of supervision and management, which is associated with farm size, is one of the major factors causing the inverse relationship between size and productivity. Digital technology, which has grown in popularity in recent years, can effectively substitute for manual labor and significantly improve farmers' monitoring and management capacities, potentially addressing the inverse relationship.
Methods: Based on data from a survey of farms in Shandong Province in 2020, this paper employs a two-stage least squares regression model to estimate the impact of herd size on dairy cow productivity and investigate how the adoption of digital technology has altered the impact of herd size on dairy cow productivity.
Results: According to the findings, there is a significant and negative impact of herd size on milk productivity for China's dairy farms. By accurately monitoring and identifying the time of estrus, coupled with timely insemination, digital technology can mitigate the negative impact of herd size on milk productivity per cow.
Discussion: To increase dairy cow productivity in China, the government should promote both small-scale dairy farming and focus on enhancing management capacities of farm operators, as well as large-scale dairy farms and increase the adoption of digital technologies.
Introduction
Population growth, rising incomes, and urbanization are driving growth in demand for livestock-derived foods across the globe, including dairy (Lagrange et al., 2015). Global cow milk production must increase in response to the rising demand for dairy products. However, dairy farming is increasingly blamed for environmental and climatic damage because it emits greenhouse gases (GHGs) (Herron et al., 2022). Improving cow productivity can be an effective strategy for sustainable milk production (Kelly et al., 2020) while reducing the associated environmental impacts (Lamkowsky et al., 2021; Faverdin et al., 2022).
Driven by rapid growth and technological innovation, global dairy farming has experienced profound structural change over the past decades, including rapid growth in the average size of primary production units and a shift toward fewer and larger firms in many countries (FAO (Food and Agriculture Organization of the United Nations), 2010). Such changes have significant consequences for farming productivity. One of the most debated findings in agricultural economics is the inverse relationship (IR) between farm size and agricultural productivity (Helfand and Taylor, 2021; Julien et al., 2021). The inverse relationship, first discovered by Chayanov during his study of the Russian peasantry (Chayonov, 1966), was subsequently detected by Sen (1962) in crop production in India. Since then, the inverse farm size-land productivity relationship has been widely discussed in academia and supported by empirical studies in many developing countries (Rada and Fuglie, 2019). IR is mainly caused by the increasing difficulty of supervision and management along with the expanding scale of the operation (Feder, 1985; Ferreira and Féres, 2020), an imperfection in market factors (Sheng et al., 2018), and measurement errors and omitted variables (Deininger et al., 2018a). Other studies have found that the inverse farm size-agricultural productivity relationship has reversed due to new technology adoption and institutional arrangements (Otsuka et al., 2016; Deininger et al., 2018b). For example, the latest breeding, tillage, and information technologies make labor supervision easier and may attenuate diseconomies of scale for large-scale farmers (Deininger and Byerlee, 2012). Moreover, coupled with the development of non-farm employment, the emergence of new institutional arrangements such as farm machinery services facilitates the substitution of machines for labor, resulting in a reversal of the inverse farm size-agricultural productivity relationship (Wang et al., 2016; Yamauchi, 2016).
Though livestock breeding and crop production differ, both confront the increasing difficulty of supervision and management as the farm size expands, but the literature is less clear on the existence of IR. Most of the extant literature on the relationship between herd size and dairy cow yield is based on the descriptive statistical analysis method, which discovered that the larger the herd size, the higher the dairy yield (Lerman, 2008; Yu, 2012; Krpalkova et al., 2016). While some studies have found that larger herd size leads to higher dairy cow yield in the United States, there is no correlation between herd size and dairy cow yield outside of the Southern and Western regions (Weersink and Tauer, 1991). Some scholars have identified that milk production decreases with an increase in herd size (Brown and White, 1973), while others have empirically analyzed the determinants of dairy cow yield and concluded that herd size, in India, has a significantly positive impact on dairy cow yield (Kumar et al., 2020). Ma et al. (2019) found that with grazing dairy farms in New Zealand, an additional increase in stocking rate increased milk solids production per hectare by between 17 and 25% but decreased milk solids production per hectare cow by between 5 and 12%. They found that milking interval, dairy breed, farm labor, access to irrigation, and farm location were all important factors that increased milk solids production. Comparisons need to adjust as much for differences between farming systems (backyard, grazing, TMR) as access to critical inputs such as irrigation. Few studies have empirically analyzed the impact of herd size on dairy cow yield in China and so have missed the opportunity to identify potential causal relationships between herd size and cow yield.
To our knowledge, the role and impact of digital technology in improving cow yield and whether it differs in impact with herd size have not been well researched. Digital technology is a product or service included in or carried by information and communication technology (Lyytinen et al., 2016; von Briel et al., 2018) and comprises two main categories: precision farming technologies and software tools (Birner et al., 2021). In the dairy industry context, digital technology is known as Precision Dairy Farming or smart agriculture technology (Werkheiser, 2018; Eastwood et al., 2019). It may influence the dairy production process in two ways: First, digital technology automates operations and streamlines production steps and labor intensity, thus reducing the demand on operators' management abilities while increasing labor productivity (Barnes et al., 2019; Dela Rue et al., 2020; Yang et al., 2021). Second, digital technology collects data and automatically generates reports (Smith, 2020) to aid operators in decision-making (Huang et al., 2018; Parikoglou et al., 2022) and to improve management efficiency. However, the adoption of digital technologies remains relatively low in dairy farms around the world (Borchers and Bewley, 2015; Gargiulo et al., 2018). The barriers to adopting digital technologies include high initial investment costs and a lack of skilled labor (Pivoto et al., 2019; Bolfe et al., 2020). Compared to small-scale farmers, larger farmers are better able to adopt digital technologies (Lambert et al., 2015; Kolady et al., 2021) due to larger farmers needing more tools to manage their more complex production systems (Carrer et al., 2022) and economies of scale (Zhang et al., 2019; Mao et al., 2021). In China, digital technologies have gained popularity in dairy production systems incorporating TMR feeding (Cox, 2007). Such technologies include automatic cup removers and automatic teat cleaning with disinfection (Edwards et al., 2015), automatic temperature and weight recording devices, milk component monitoring and milk conductivity indicators (Bewley, 2010; Eastwood et al., 2012), wireless identification devices, automatic farm management software (Eastwood et al., 2012) and cow estrus detection tools (Mayo et al., 2019). Except for cow estrus detection, other technologies have either a high or low penetration rate among farms of various sizes (Dong, 2017; Li, 2017; Peng and Li, 2020) with little observed impact on the relationship between herd size and milk yield. Chinese farms of different sizes diverge in their adoption of estrus detection technology, which is more prevalent among farms of more than 1,000 heads and less popular on farms of less than 1,000 heads (Peng and Li, 2020).
Furthermore, cow estrus monitoring is a crucial activity in dairy farming. In the event of a missed estrus, farmers require another estrous cycle (~21 days), which delays the conception of the cows and, consequently, their milk supply (Gaude et al., 2021). Currently, methods for monitoring estrus in cows include manual and automated inspection. Manual inspection is labor-intensive, and a lack of management leads to missed estrus. Automatic estrus detection, on the other hand, is a type of wearable information monitoring technology in which sensors like pedometers and collars are worn on the legs or necks of cows. The daily step data collected by the sensors is automatically obtained via signal receivers and sent to computer software, which then performs statistical analysis to build an information system for dynamic monitoring of cow estrus. Implementing automatic estrus detection in dairy cows can assist management in improving the detection rate and reduce the incidence of missed estrus (Rorie et al., 2002; Steeneveld et al., 2015).
Therefore, this paper aims to extend previous research by analyzing the impact of herd size on dairy cow productivity in China and take estrus detection technology as an example to explore the impact of the interaction between herd size and digital technology adoption on dairy cow productivity. China is an interesting case for two reasons. First, driven by the “Dual Circulation” strategy (Lin and Wang, 2021; Guo et al., 2022), the Chinese dairy sector needs a new focus to meet the strong domestic demand for dairy products, with domestic production as the mainstay and domestic and international supply reinforcing each other. According to the forecast of China's Ministry of Agriculture and Rural Affairs, China's cow milk production and dairy consumption will reach 43.89 million tons and 69.33 million tons by 2030, with a production-demand gap of 25.44 million tons (MEWEC (Market Early Warning Expert Committee, Ministry of Agriculture and Rural Affairs), 2021). Domestic milk production would need to be boosted by 58% to meet the “Dual Circulation” requirements. Second, there are two ways to increase domestic milk production. One approach is to increase the stock of dairy cows.
From 2010 to 2020, the dairy cow numbers fell from 12.108 million heads to 10.43 million heads (HF (Holstein Farmer), and DC (dairy consultants), 2021). The main reason is that China's dairy production is shifting from backyard farming to larger-scale dairy farm production. Thus, despite the considerable decline in dairy cow stock, the average size of dairy farms is expanding significantly. From 2010 to 2020, the average number of dairy cows farmed in China increased from 5.24 to 20.37 head of stock/ dairy farming households at an average annual growth rate of 14.54% (CAHVYED (China Animal Husbandry Veterinary Yearbook Editorial Department), 2021). The other approach is to increase dairy cow productivity. In recent years, China's cow milk production growth has primarily depended on annual cow productivity increases.
Nevertheless, a gap remains in the level of dairy cow yield between China and developed countries. In 2020, China's dairy cow yield was 8.3 tons/year, compared to 10.785 tons/year in the United States, 11.924 tons/year in Israel, and 10.702 tons/year in Canada (HF (Holstein Farmer), and DC (dairy consultants), 2021) in housed, intensive, total mixed ration (TMR) dairy systems. Cow milk production is affected by genetic and managerial factors (Norring et al., 2012; Kato et al., 2022) and the system adopted. Chinese dairy farms have primarily introduced the world-recognized high-yielding breed, the Holstein, so the discrepancy between Chinese dairy yield and that of dairy-developed countries mainly stems from gaps in management among other aspects all else being equal.
This study makes the following marginal contributions: first, while most studies have focused on the impact of farm size on crop production (Aragón et al., 2022), few have paid attention to dairy farming in China (Xia et al., 2020). The negative relationship in crop plantation stems primarily from management supervision capacity and labor effort decrease as farm size increases (Feder, 1985; Ma et al., 2022), whereas dairy farming demands more refined management than crop production. This paper takes dairy farming as an example to fill the existing research gap. Second, it explores the impact of digital technology on the herd size-dairy cow productivity relationship, providing a new perspective for increasing dairy cow yield in China. Third, it empirically tests the impact of herd size on dairy cow productivity using the two-stage least squares(2SLS) regression model in an attempt to improve the accuracy and reliability of the estimation of the impact. In addition, it employs the quantile instrumental variable method for robustness testing.
Materials and methods
Research background
Changes in the size and structure of dairy production and dairy cow yield in China
Before the 1980s, dairy farming in China was concentrated in state-owned farms, with a breeding scale of about 1,000 heads (DAC (Dairy Association of China), 2002). With the development of the market economy and relevant policies, backyard farming evolved into the main pattern of dairy farming in China and remained unchanged for some time. After the Melamine incident in 2008, the Chinese government directed dairy farming toward large-scale, standardized, and intensive development, thereby changing the dominant production model of China's dairy sector (Mo et al., 2012). By 2016, the proportion of farming on a particular scale with an annual stock of more than 100 head exceeded 50%, indicating that the proportion of large-scale dairy farming in China surpassed that of backyard farming for the first time. After 2016, farming on a certain scale became China's primary production model for dairy farming. From 2016 to 2020, the proportion of dairy farms with an annual stock of more than 100 heads grew from 52.3 to 67.2% (DAC (Dairy Association of China), 2021), with an average annual growth rate of 6.9%.
China's dairy cow yield has also witnessed growth as the country's dairy cow systems evolved from backyard-oriented to a large-scale operation. During the 2008–2020 period, the dairy cow yield grew from 4.575 tons/year to 8.3 tons/year nationwide, registering an average annual growth rate of 5.09%. Since 2004, the Ministry of Agriculture has classified dairy farming modes into four categories/systems by the average annual stock of dairy cows: (1) backyard breeding of a stock of fewer than ten heads; (2) small-scale breeding of an annual stock of 10–50 heads; (3) medium-scale breeding of an annual stock of 51–500 heads; and (4) large-scale breeding of an annual stock greater than 500 heads. From 2008 to 2020, dairy cow production of different scales registered yield growth: (1) The average yield of backyard dairy cows increased from 5.14 to 5.48 tons/year with an average annual growth rate of 0.54%; (2) The average yield of small-scale dairy cows increased from 5.16 to 5.61 tons/year with an average annual growth rate of 0.7%; (3) The average yield of medium-scale cows increased from 5.56 to 6.66 tons/year with an average annual growth rate of 1.52%; (4) The average yield of large-scale cows increased from 6.35 to 8.17tons/year, with an average annual growth rate of 2.12% (PDNDRC (Price Department of National Development Reform Commission), 2021).
Shandong is one of the most critical dairy farming provinces in China. From 2016 to 2020, cow milk production in this province occupied about 7% of the total milk nationwide and ranked fourth in China, and its dairy cows accounted for about 8.5% of the national stock and ranked fifth in the country. In the past five years, the dairy herd size and cow yield have been improving rapidly in the province. The proportion of farms with more than 100 heads showed an average annual growth rate of 31.57%, ranking fourth nationwide, and the average annual growth rate of dairy cow yield was 15.04%, ranking second across China.
Use of estrus detection technology in dairy cows
The reproductive performance of dairy cows is critical to the profits of a farm because it affects the time interval between the parity of cows, which in turn affects cow milk production (Reith and Hoy, 2018). The estrus cycle of Holsteins generally lasts 18–23 days, with 21 days on average. The duration of estrus within each cycle is short, lasting 1.7–30.7 h (Dobson et al., 2008). Cows begin to produce milk only after timely and accurate detection of cows in estrus, timely breeding, the conception of cows, and successful delivery of calves. Farmers need to wait for the next estrus cycle (21 days on average) if they fail to detect the estrus, and the increased inputs during cows' missed conception will result in the farm incurring economic losses. Therefore, detecting estrus is a crucial management factor affecting the reproductive performance of dairy cows (Dolecheck et al., 2015; Endo, 2022).
Historically, cow estrus monitoring was accomplished mainly through manual labor, such as external observations of cow activity and rectal and vaginal examinations. These methods, which rely on the experience of farmers, are time-consuming and labor-intensive with a high human cost. In addition, cow estrus frequently occurs at night, when observers are most fatigued, making manual monitoring difficult and possibly resulting in missed estrus. Manual monitoring has become even more challenging as herd sizes grow, with detection accuracy often falling below 50% (Roelofs et al., 2010). Since then, automatic estrus detection has gradually replaced manual detection (Homer et al., 2013). Automatic estrus detection is designed on the principle that cow activity increases when a cow is in estrus. The adult cow will wear a pedometer to identify its number and track its movement. When approached by a cow, the sensor automatically collects data about it and transmits it to a computer, which sends the movement data to a computerized estrus monitoring and analysis system to generate a report. Breeders schedule timely breeding according to the estrus report form. Pedometer estrus monitoring can be as accurate as 80%−90% or even 100% (Stevenson et al., 2014).
Small and medium-sized farms in China still detect cow estrus manually, whereas large-scale farms have utilized cow estrus monitoring systems (Liu et al., 2019). The Chinese self-designed automated estrus monitoring system is still in its early stages, and companies such as Afimilk and SCR from Israel, Delaval from Sweden, and Nadap from The Netherlands have all developed market-ready products (Cao et al., 2013). However, the imported estrus monitoring systems are costly and necessitate specific computer skills on the part of the breeders (Luo et al., 2019).
Econometric model
To examine the impact of herd size on dairy cow yield, we developed the following econometric model.
In model (1), we denote cow yield by the explained variable yi, referred to as daily milk production per cow for farm i. The sizei is herd size, referring to a farm's total cow numbers. Xi refers to other factors that influence cow yield. We choose basic characteristics of operators and farming features as control variables based on extant research. These include the operator's age; years of education; years of farming; gender; the proportion of hired workers, expressed as the ratio of the number of hired workers to the total number of workers; fixed asset input; feed input per cow; farming density; cow parity; breed, expressed as the proportion of the number of Holsteins to the entire number of cows; the economic region where the farm is located. The εi in Equation (1) is a random disturbance term whereas β0, β1 and γ are model parameters to be estimated. Our focus is β1,and a negative and statistically significant β1would suggest the existence of an inverse herd size-dairy cow yield relationship.
To further test the role of digital technology on the impact of herd size on dairy cow yield, this paper creates an interaction term of herd size and whether digital technology is applied based on model (1) and constructs the model as follows.
In model (2), DTi is a dummy variable for ‘whether digital technology is applied'. Value is taken as 1 when digital technology is applied and 0 when not applied. μi is a random disturbance term. α0, α1, α2, α3and δ are model parameters to be estimated. α1, α2, α3are the parameters of our interest. A positive and statistically significant α2 indicates the dairy cow yield gap between small and larger farms diminishes when digital technology is used.
The key explanatory variables of herd size in models (1) and (2) are theoretically endogenous variables that may lead to endogenous problems and estimate bias in the model. We use the two-stage least squares (2SLS) regression model to solve endogenous problems. We chose the “average size of county-level farms in 2017” as the instrumental variable. This variable meets the necessary condition for being an instrumental variable for the herd size: the average size of county-level farms in 2017 directly influences the size of a single farm in the county in 2020 but does not directly affect the dairy cow yield on a single farm in the county. In equation (2), for the interaction term between digital technology and herd size, we take the interaction term between digital technology and “average size of county-level farms in 2017” as the instrumental variable. In the empirical process, the model underwent the endogenous test and the validity test of the instrumental variables.
To ensure the reliability of the estimation, we adopt two methods for the robustness tests. The first is to change the dependent variable. We transformed the dependent variable of the average daily cow yield in 2020 into the average daily cow yield in spring and winter in 2020. That is because cows dislike heat, and milk production is generally low in summer and autumn, while the average daily yield is high in spring and winter. The second is to change the estimation method. We use the instrumental variable quantile regression method.
Data sources and variable definitions
This paper incorporates data from a survey of dairy farms in Shandong Province in 2020 for empirical research. Due to the dispersed distribution of dairy farms in each county, we gathered the managers/owners of dairy farms to the animal husbandry station of each county and recruited the trained graduate students as enumerators to conduct one-on-one interviews with them. The interview lasted about an hour, and the enumerators filled out questionnaires covering the basic characteristics of the farm manager/owner as well as the farm's cost and benefits in 2019 and 2020, including total milk production, price and quantity of milk sold, cost of feeding the entire herd of cows, amount of feed input, depreciation of fixed assets, labor input and adoption of digital technology, in particular estrus detection technology.
We first used stratified random sampling in the survey and selected 15 cities with relatively more large-scale dairy farming households, considering regional distribution and development disparities. Then, based on the number of dairy farming households of each county in the 15 cities, we selected 1–5 counties with more farming households and conducted a census of dairy farming households in each of these counties. The 15 cities selected in Shandong Province are shown in Figure 1. Zaozhuang is excluded due to relatively few dairy farmers in the city. The survey obtained a total of 361 samples, of which 324 were valid, accounting for 89.75% of all samples.
The most important three variables used in this analysis are cow yield, herd size, and adoption of digital technology. Cow yield is measured in daily milk production per cow reported by farm manager/owner. We define herd size as the highest number of cows per farm during the year. Many studies use the number of milking cows to define herd size (Huettel and Jongeneel, 2011; Dong et al., 2016). However, this is not the case in China. Our definition of herd size follows China Agricultural Product Cost-Benefit Complication, published by the National Development and Reform Commission. The adoption of digital technology is a dummy variable equal to one if the farm adopts dairy cow estrus detection technology and zero otherwise, directly reported by manager/owner. The analysis also controls for a large number of farm characteristics. The labor input includes hired labor and family labor expressed as daily costs per cow. The cost of family labor is reported by the farm manager/owner. The proportion of hired workers and breeds are expressed in percentages. Fixed assets input includes total mixed ration (TMR), milking machines, feeding machines and some transport machines, measured by the depreciation. Education is expressed as the manager's years of school and farming experience as the number of years the manager has raised dairy cows. We define breeding density as the total raising land area divided by total cow numbers. The amount of concentrated feed is expressed in quantity. Parity is expressed as the average years the milking cows used. We also controlled the regional difference and expressed in dummy variables.
The definitions and descriptive statistics of the variables used in this paper are shown in Table 1. On average, the milk production per cow is 28.119 kg /day. The average herd size is 580 cows. 25.8% of dairy farms have adopted digital technology, 76.3% have hired workers, and 90.1% of the operators are male. The operators' average age, education level and dairy farming experience are 47.912 years of age, 10.766 years of education and 15.207 years of dairy farming, respectively. In terms of expenditures, the daily depreciation of fixed assets per cow is 0.774 yuan (0.112 US dollars; 2019 dollars). In terms of variable costs, a milking cow receives 9.75 kg of concentrate feed per day. Furthermore, the daily wage for employed and family laborers is 3.472 yuan per cow. The land area per cow is 0.178 Mu (1 Mu = 1/15th of a hectare). The average parity of the cows is 3.893 gestations per cow, and 96.1% of dairy farms use Holstein cows. Furthermore, 32.9% of the farms are located in the central area, 8.5% in the western area, and 58.6% in the Jiaodong area.
Results and discussion
The impact of herd size on the dairy cow yield
Column (1) in Table 2 reports the ordinary least squares (OLS) estimation results of model (1). The coefficient of Herd size is positive and significant at a 1% level, and a 1% increase in herd size increases the dairy cow yield by 3.2%, suggesting that small farms will increase dairy cow yield by enlarging their herd size in China. The coefficients on the adoption of digital technology (estrus detection technology), Proportion of hired workers, Years of education, fixed asset input, and labor input are all positive and significant, indicating that implementing these practices could also increase dairy cow yield. The coefficient of breeding density is significantly negative, consistent with Ma et al. (2019). In addition, dairy cow yields were higher on farms in the western and Jiaodong areas.
It should be noted that there is a causal relationship between herd size and cow yield but that using the OLS estimation may lead to biased results. Therefore, we used the two-stage least squares (2SLS) regression model. Before doing so, we first tested the validity of the instrumental variables. The Hausman test results reported that the null hypothesis is rejected at a 1% level of significance, indicating that the herd size is considered an endogenous variable. We further conducted weak instrumental variable tests. The Cragg-Donald Wald F-statistic was 14.527, significantly greater than the threshold for the weak instruments test formalized by Stock and Yogo (2005). The above results proved that the model does not have a weak instrumental variable problem. Therefore, the instrumental variable selected in this paper, namely “average size of county-level farms in 2017”, is reasonable.
Column (2) of Table 2 presents the first stage regressions of the 2SLS model (1). The estimated effect of the average size of county-level farms in 2017 on herd size is positive and significant. This implies that the larger the average size of county-level farms in 2017, the larger the herd size. As for the magnitude, a 1% increase in average size of county-level farms in 2017 is associated with a 0.181% increase in herd size.
Column (3) of Table 2 reflects the second stage estimation results of 2SLS model (1), which reveal that herd size contributes negatively and statistically significantly to dairy cow yield. When controlling for other factors, a 1% increase in herd size reduced the dairy cow yield by a 19.7%. This indicates that under current conditions where technology, fixed input, and factor input are constant, expanding herd size will result in a decline in the average dairy cow yield in China. That is because management ability and practices are primary milk production determinants (Bewley et al., 2001b). Most large-scale farms evolved from small-scale farms in China, while the large-scale farmers' managerial ability did not improve simultaneously (Hu et al., 2019). In recent years, dairy cow farming has scaled up very quickly in China, while dairy farmers have also not improved their management capacity despite expanding herd size (Liu et al., 2018), resulting in farms growing in size but attenuating their cow productivity. This is consistent with previous findings, suggesting that milk production decreases with an increase in herd size (Brown and White, 1973). The drop in milk production is greatest for those rapidly expanded farms. The reasons for this drop included lack of production ability and incorrect management practices (Speicher et al., 1978). In addition, other control variables which significantly impact dairy cow yield include the adoption of digital technology, the proportion of hired workers, years of education, breeds and the economic region where the farm is located still present a positive and significant influence on dairy cow yield. Breeding density has a negative and significant influence on dairy cow yield.
Moderating effect of digital technology
To verify that the adoption of digital technology reduces the demand for management capacity and improves management efficiency, as well as to mitigate the inverse herd size-cow yield relationship further, we empirically analyze the impact of technology adoption on the herd size-cow yield relationship based on model (2). The estimated results are presented in Tables 3, 4. Table 3 reports the OLS estimation results, which indicate that herd size positively contributes to dairy cow yield.However, according to these results, neither digital technology adoption has a significant impact on the dairy cow yield nor does the adoption of digital technology significantly impact the herd size-dairy yield relationship. To address the endogenous problem of the model, we further develop a two-stage least squares (2SLS) regression model. Before that, it was necessary to pass the endogeneity test of herd size and the weak instrumental variable test. The Hausman test results showed that the null hypothesis is rejected at a 1% significance level; the Cragg-Donald Wald F-statistic was 7.069, significantly higher than the threshold of the Stock-Yogo weak instrumental variable. According to the results of the above tests, the herd size is an endogenous variable, and the instrumental variables selected in this paper are reasonable.
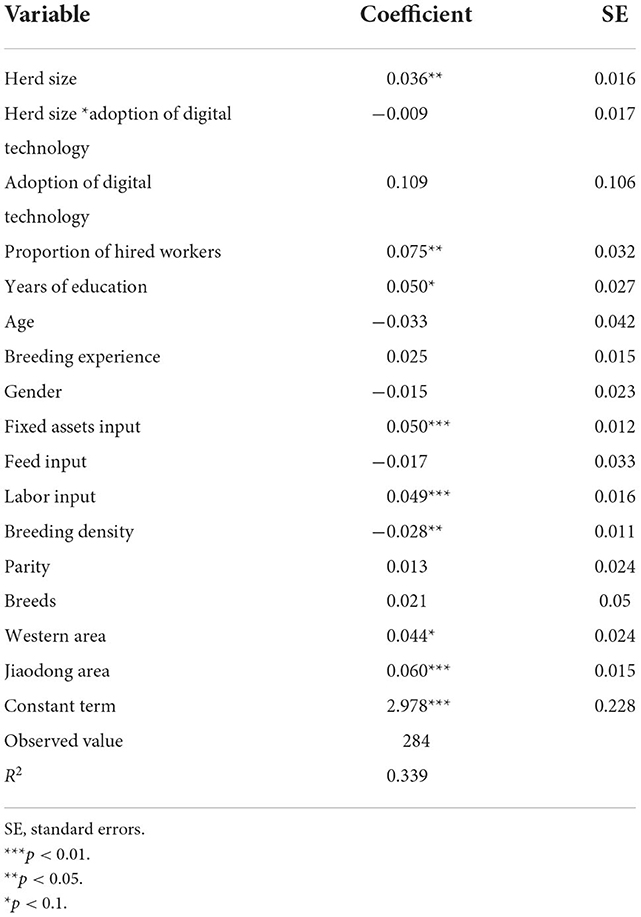
Table 3. Empirical results on the impact of digital technology adoption on the relationship between herd size and dairy cow yield by OLS.
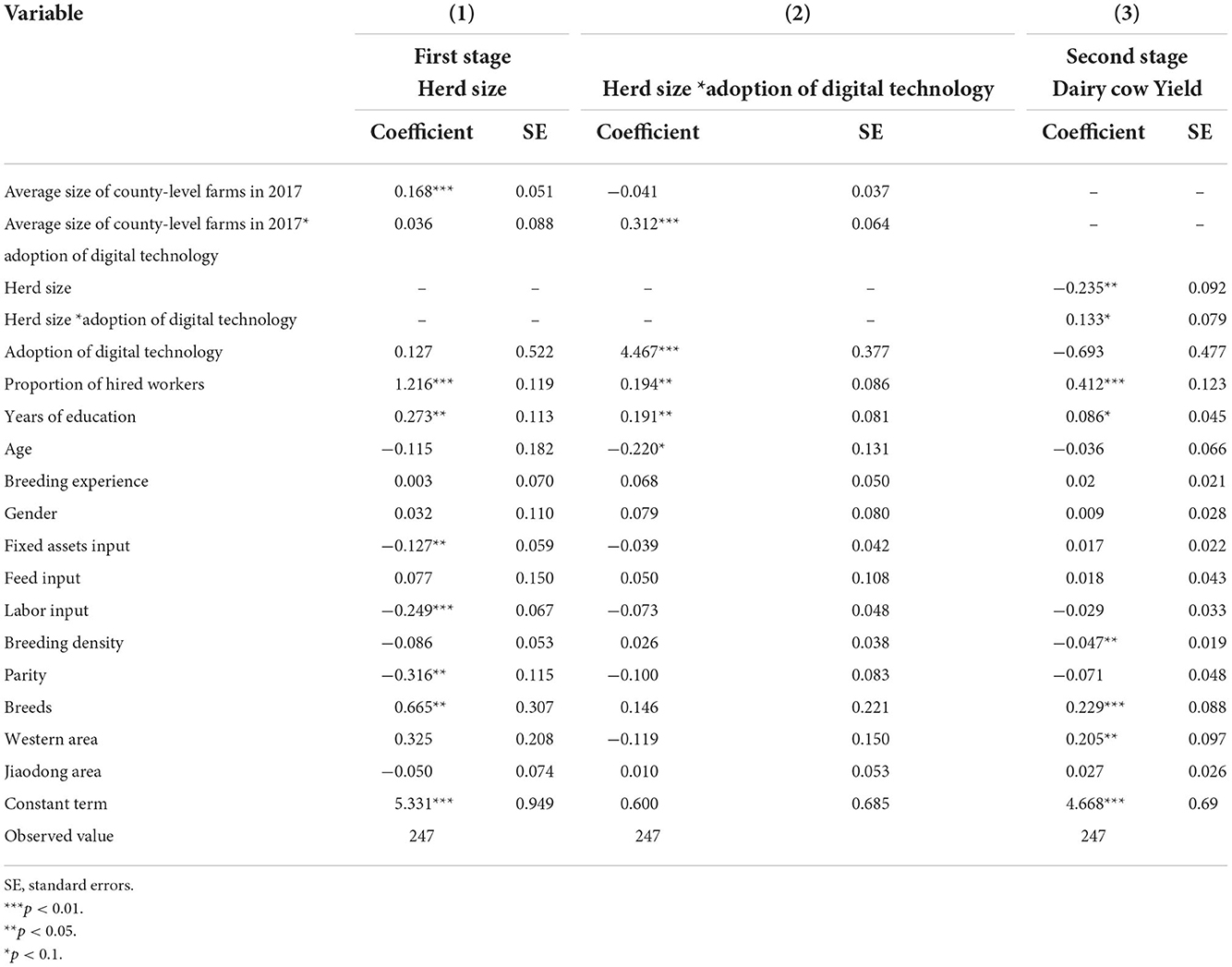
Table 4. Empirical results on the impact of digital technology adoption on the relationship between herd size and dairy cow yield by 2SLS.
Columns (1) and (2) in Table 4 report the results for the first stage regression [model (2)]. Column (1) shows that the average size of county-level farms in 2017 positively and significantly impacts herd size. Column (2) shows a positive and significant effect of the interaction between the average size of county-level farms in 2017 and the adoption of digital technology on the interaction between herd size and adoption of digital technology. The results suggest that the average size of county-level farms in 2017 increased the herd size.
Table 4 column (3) reports the second stage estimates of the 2SLS model (2). The regression shows that herd size significantly negatively affects dairy cow yield, and 1% increase in herd size leads to a reduction of 23.5% in dairy cow yield. The negative impact of herd size on dairy cow yield diminishes with the adoption of digital technology. As mentioned above, the reason for the negative effect of herd size on dairy cow yield is that dairy farmers have expanded herd size but have not improved their managerial ability. However, as herd size increases, herd management is the biggest challenge (Bewley et al., 2001a). The adoption of digital technology can help to reduce the requirements for managerial ability and improve managerial efficiency (Eastwood et al., 2012, 2016; Cabrera et al., 2020). Farmers operating larger farms are more likely to adopt digital technology (Läpple et al., 2015; Min et al., 2020) to take advantage of economies of scale (Pierpaoli et al., 2013; Tamirat et al., 2018). Therefore, the adoption of digital technology can attenuate the negative impact of herd size on dairy cow yield. This is supported by the literature, which indicates that larger farms adopting new technologies or management practices can increase milk production (Khanal et al., 2010) due to a scale-bias toward technology adoption (Abeni et al., 2019). China is experiencing a rapid digital transformation of agriculture (Cui et al., 2022; Shen et al., 2022). Large-scale farms have advantages in adopting digital technology (Xie et al., 2021). These suggest that the impact of herd size on dairy milk yield may change as China transforms.
Robustness tests
Tables 5, 6 present the results of the two-stage least squares (2SLS) regressions for the first robustness tests. Column (1) of Table 5, and columns (1) and (2) of Table 6 report the first-stage estimation results. Column (1) of Table 5 and column (1) of Table 6 shows that the average size of county-level farms in 2017 positively and significantly impacts herd size. Column (2) of Table 6 shows that the interaction between average size of county-level farms in 2017 and the adoption of digital technology has a positive and significant impact on the interaction between herd size and the adoption of digital technology. These results are consistent with the above results, indicating that our results are robust.
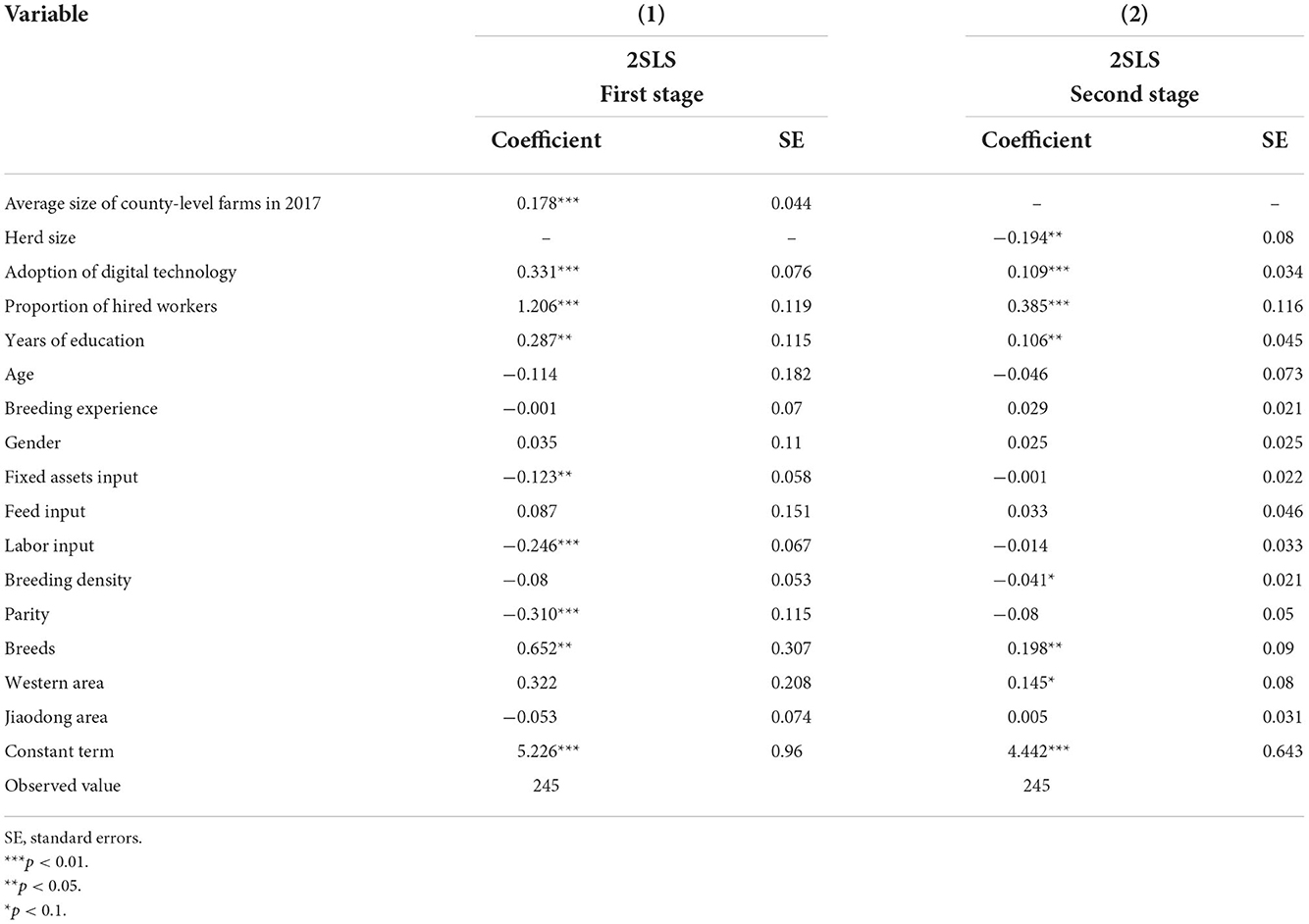
Table 5. Robustness test: the impact of herd size on the dairy cow yield by change the dependent variable.
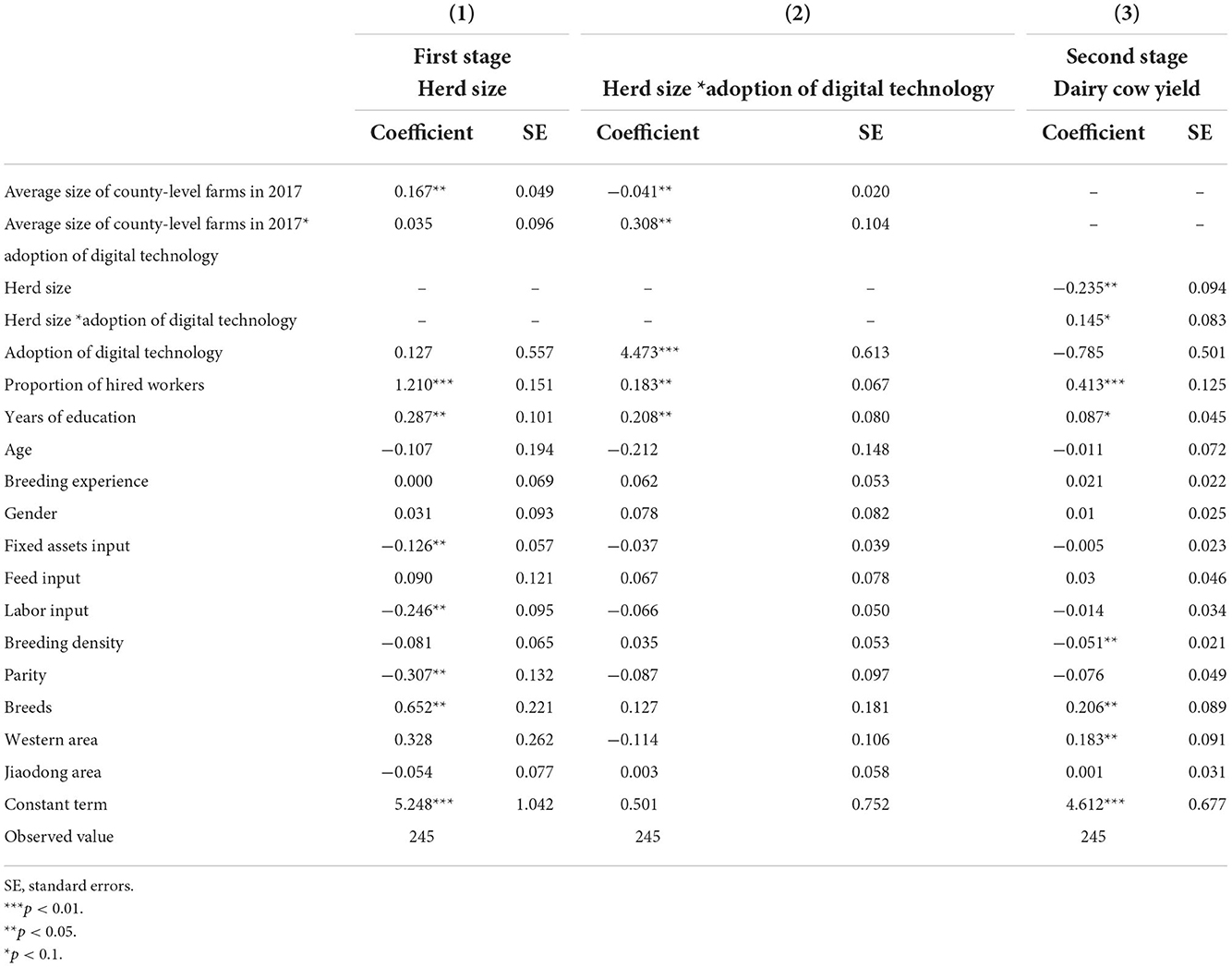
Table 6. Robustness test: the impact of digital technology adoption on the relationship between herd size and dairy cow yield by change the dependent variable.
The regression result in column (2) of Table 5 shows significantly negative coefficients for herd size, implying that statistically significant negative impact of herd size on dairy cow yield corroborating the regression results above and indicating that the research conclusions are robust. The regression result in column (3) of Table 6 shows that herd size significantly influences dairy cow yield, with digital technology significantly attenuating the negative impact of herd size on dairy cow yield, which is consistent with the previous empirical results, indicating the robustness of our findings. In addition, we performed an endogeneity test of herd size and the weak instrumental variable test. The results of the Hausman test indicated that the null hypotheses should be rejected for all models. The Cragg-Donald Wald F-statistic exceeds the threshold for the weak instrumental variable test formalized by Stock and Yogo, indicating our estimations do not suffer from weak instrumental variable problems.
Tables 7, 8 present the estimation results for the second robustness test. Table 7 reports the regression results of the quantile instrumental variables method for the influence of herd size on dairy cow yield. The impact of herd size on dairy cow yield is significantly negative at different quantiles, once again confirming that the main results of this paper are robust. In addition, Table 8 reports the regression results of the quantile instrumental variable method for the influence of digital technology adoption on herd size and dairy cow yield. First, the regression results show that herd size has a significantly negative impact on dairy cow yield, and the adoption of digital technology can significantly mitigate the negative impact of herd size on dairy cow yield, indicating that the above research findings are robust. Second, the regression coefficient of the interaction term of herd size and technology adoption was the largest at the 75th quantile and showed a increasing trend as the quantile increased, indicating that the digital technology adoption on farms with high cow yield had a greater mitigating effect on the negative impact of herd size on dairy cow yield.
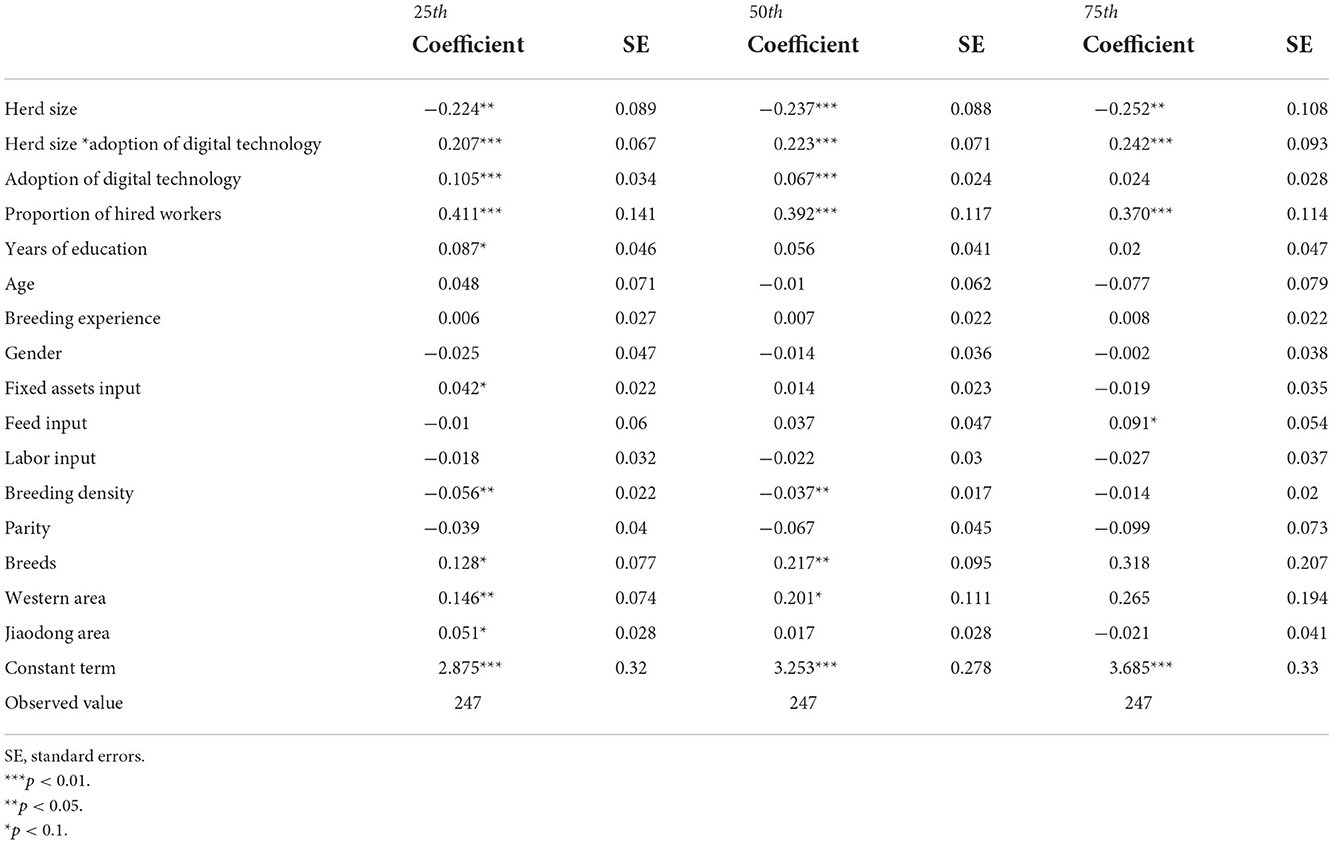
Table 8. Robustness test: Influence of digital technology adoption on the relationship between herd size and dairy cow yield at different quartiles.
Conclusions
Increasing cow milk productivity is essential for ensuring sustainable milk production. However, the impact of herd size on milk productivity is complicated. Based on research data from dairy farms of certain scales in Shandong Province in 2020, this paper used a two-stage least squares (2SLS) regression model to explore the influence of herd size on dairy cow yield and further discussed the impact of digital technology adoption on the herd size-dairy cow yield relationship, citing dairy cow estrus monitoring technology as an example. The main findings are as follows: first, herd size significantly negatively impacts dairy cow yield; second, the adoption of digital technology can attenuate the negative impact of herd size on dairy cow yield.
According to the findings of this paper, our estimates are in line with previous studies that found a negative influence of farm size on land productivity in developing countries. However, the government has provided a series of large-scale oriented subsidies for dairy farms since 2008, resulting in the rapid development of scale in China's dairy sector. The large-scale farms that grew fast from small-scale farms have not upgraded their management and other aspects, resulting in stagnation in China's milk production. Thus, a possible policy option would be to promote small-scale dairy farming to enhance dairy cow yield. Furthermore, the results of this paper also show that the adoption of digital technology can mitigate the negative impact of herd size on dairy cow yield. This is consistent with extant studies that new technologies may change the negative influence of farm size on land productivity. It means large-scale farms' managerial ability could be offset by adopting digital technologies. The role of digital technologies in improving dairy yield is important. As a result, the government should encourage large-scale farms rapidly expanding from small-scale farms to use digital technologies to boost their dairy cow yields. Further research suggestions prompted from the conclusion of this paper include using continuous multi-period panel data to explore the impact of herd size on dairy cow yield. This paper uses cross-sectional data, so the author will extend this work and conduct a follow-up survey on dairy farms to analyze the dynamic influence of herd size on dairy cow yield. Also, while estrus monitoring was chosen as an example for digital technology, the influence of the adoption of different digital technologies on the herd size-cow yield relationship can be compared in further studies.
Data availability statement
The original contributions presented in the study are included in the article/supplementary material, further inquiries can be directed to the corresponding authors.
Author contributions
Conceptualization and investigation: YQ and JH. Data curation, formal analysis, methodology, visualization, and writing—original draft: YQ. Funding acquisition, project administration, and resources: JH. Supervision: JH and NS. Validation: NS and QZ. Writing—review and editing: JH, NS, and QZ. All authors contributed to the article and approved the submitted version.
Funding
This research was supported by the National Natural Science Foundation of China (71973066) and the Fundamental Research Funds for Central Universities (SKQY2020002).
Acknowledgments
The authors acknowledge the sponsorships of the National Natural Science Foundation of China (71973066) and the Fundamental Research Funds for Central Universities (SKQY2020002) under the Priority Academic Program Development of Jiangsu Higher Education Institutions (PAPD).
Conflict of interest
The authors declare that the research was conducted in the absence of any commercial or financial relationships that could be construed as a potential conflict of interest.
Publisher's note
All claims expressed in this article are solely those of the authors and do not necessarily represent those of their affiliated organizations, or those of the publisher, the editors and the reviewers. Any product that may be evaluated in this article, or claim that may be made by its manufacturer, is not guaranteed or endorsed by the publisher.
References
Abeni, F., Petrera, F., and Galli, A. (2019). A survey of Italian dairy farmers' propensity for precision livestock farming tools. Animals 9, 202. doi: 10.3390/ani9050202
Aragón, F. M., Restuccia, D., and Rud, J. P. (2022). Are small farms really more productive than large farms? Food Policy 106, 102168. doi: 10.1016/j.foodpol.2021.102168
Barnes, A. P., Soto, I., Eory, V., Beck, B., Balafoutis, A., Sánchez, B., et al. (2019). Exploring the adoption of precision agricultural technologies: a cross regional study of EU farmers. Land Use Policy 80, 163–174. doi: 10.1016/j.landusepol.2018.10.004
Bewley, J., Palmer, R. W., and Jackson-Smith, D. B. (2001a). An overview of experiences of Wisconsin dairy farmers who modernized their operations. J. Dairy Sci. 84, 717–729.doi: 10.3168/jds.S0022-0302(01)74526-2
Bewley, J., Palmer, R. W., and Jackson-Smith, D. B. (2001b). Modeling milk production and labor efficiency in modernized wisconsin dairy herds. J. Dairy Sci. 84, 705–716. doi: 10.3168/jds.S0022-0302(01)74525-0
Bewley, J. M. (2010). “Precision dairy farming: advanced analysis solutions for future profitability,” in Paper presented at the in Proc. 1st North Am. Conf., Precis. Dairy Manag. Toronto, Canada.
Birner, R., Daum, T., and Pray, C. (2021). Who drives the digital revolution in agriculture? A review of supply-side trends, players and challenges. Appl. Econ. Perspect. Policy 43, 1260–1285. doi: 10.1002/aepp.13145
Bolfe, É. L., Jorge, L. A. D. C., Sanches, I. D. A., Luchiari Júnior, A., DA Costa, C., Victoria, C. C., et al. (2020). Precision and digital agriculture: adoption of technologies and perception of Brazilian farmers. Agriculture 10, 653. doi: 10.3390/agriculture10120653
Borchers, M. R., and Bewley, J. M. (2015). An assessment of producer precision dairy farming technology use, prepurchase considerations, and usefulness. J. Dairy Sci. 98, 4198–4205. doi: 10.3168/jds.2014-8963
Brown, C. A., and White, J. M. (1973). Immediate effects of changing herd size upon milk production and other dairy herd improvement measures of management. J. Dairy Sci. 56, 799–804. doi: 10.3168/jds.S0022-0302(73)85252-X
Cabrera, V. E., Barrientos-Blanco, J. A., Delgado, H., and Fadul-Pacheco, L. (2020). Symposium review: real-time continuous decision making using big data on dairy farms. J. Dairy Sci. 103, 3856–3866. doi: 10.3168/jds.2019-17145
CAHVYED (China Animal Husbandry and Veterinary Yearbook Editorial Department). (2021). China Animal Husbandry and Veterinary Yearbook. Beijing: China Agriculture Press. (in Chinese).
Cao, X., Huang, S., Feng, S., Xue, C., Chang, Z., Wang, Z., et al. (2013). Applications of activity monitoring technology in dairy cattle breeding management. China Dairy Cattle 8, 37–40. doi: 10.3969/j.issn.1004-4264.2013.08.008 (in Chinese).
Carrer, M. J., Filho, H. M. D. S., Vinholis, M. D. M. B., and Mozambani, C. I. (2022). Precision agriculture adoption and technical efficiency: an analysis of sugarcane farms in Brazil. Technol. Forecast. Soc. Change 177, 121510. doi: 10.1016/j.techfore.2022.121510
Cox, B. G. (2007). Impact of Precision Feeding Strategies on Whole Farm Nutrient Balance and Feeding Management [Dissertation/Master's Thesis]. Blacksburg (Virginia): Virginia Polytechnic Institute and State University.
Cui, M., Qian, J., and Cui, L. (2022). Developing precision agriculture through creating information processing capability in rural China. J. Rural Stud. 92, 237–252. doi: 10.1016/j.jrurstud.2022.04.002
DAC (Dairy Association of China). (2002). China Dairy Yearbook. Beijing: China Agriculture Press. (in Chinese).
DAC (Dairy Association of China). (2021). China Dairy Quality Report. Beijing: China Agriculture Press. (in Chinese).
Deininger, K., and Byerlee, D. (2012). The rise of large farms in land abundant countries: do they have a future? World Dev. 40, 701–714. doi: 10.1016/j.worlddev.2011.04.030
Deininger, K., Jin, S., Liu, Y., and Singh, S. K. (2018a). Can labor-market imperfections explain changes in the inverse farm size?productivity relationship? Longitudinal evidence from rural India. Land Econom. 94, 239–258. doi: 10.1016/S0305-750X(03)00101-3
Deininger, K., Nizalov, D., and Singh, S. (2018b). Determinants of productivity and structural change in a large commercial farm environment: evidence from ukraine. World Bank Econ. Rev. 32, 287–306. doi: 10.1093/wber/lhw063
Dela Rue, B. T., Eastwood, C. R., Edwards, J. P., and Cuthbert, S. (2020). New Zealand dairy farmers preference investments in automation technology over decision-support technology. Anim. Prod. Sci. 60, 133–137. doi: 10.1071/AN18566
Dobson, H., Walker, S. L., Morris, M. J., Routly, J. E., and Smith, R. F. (2008). Why is it getting more difficult to successfully artificially inseminate dairy cows? Animal 2, 1104–1111. doi: 10.1017/S175173110800236X
Dolecheck, K. A., Silvia, W. J., Heersche, G., Chang, Y. M., Ray, D. L., Stone, A. E., et al. (2015). Behavioral and physiological changes around estrus events identified using multiple automated monitoring technologies. J. Dairy Sci. 98, 8723–8731. doi: 10.3168/jds.2015-9645
Dong, F., Hennessy, D. A., Jensen, H. H., and Volpe, R. J. (2016). Technical efficiency, herd size, and exit intentions in U.S. Dairy farms. Agr. Econ.-Blackwell. 47, 533–545. doi: 10.1111/agec.12253
Dong, X. (2017). Weigh-in-Motion of the Dairy Cow Based on STM32 Microcontroller [Dissertation/Master's Thesis]. Tai'an (Shandong): Shandong Agricultural University (in Chinese).
Eastwood, C., Klerkx, L., Ayre, M., and Dela Rue, B. (2019). Managing Socio-Ethical challenges in the development of smart farming: from a fragmented to a comprehensive approach for responsible research and innovation. J. Agric. Environ. Ethics 32, 741–768. doi: 10.1007/s10806-017-9704-5
Eastwood, C. R., Chapman, D. F., and Paine, M. S. (2012). Networks of practice for co-construction of agricultural decision support systems: case studies of precision dairy farms in Australia. Agr. Syst. 108, 10–18. doi: 10.1016/j.agsy.2011.12.005
Eastwood, C. R., Jago, J. G., Edwards, J. P., and Burke, J. K. (2016). Getting the most out of advanced farm management technologies: roles of technology suppliers and dairy industry organisations in supporting precision dairy farmers. Anim. Prod. Sci. 56, 1752. doi: 10.1071/AN141015
Edwards, J. P., Dela Rue, B. T., and Jago, J. G. (2015). Evaluating rates of technology adoption and milking practices on New Zealand dairy farms. Anim. Prod. Sci. 55, 702–709. doi: 10.1071/AN14065
Endo, N. (2022). Possible causes and treatment strategies for the estrus and ovulation disorders in dairy cows. J. Reprod. Dev. 68, 85–89. doi: 10.1262/jrd.2021-125
FAO (Food Agriculture Organization of the United Nations). (2010). The State of Food and Agriculture: Livestock in the Balance. Available online at: https://www.fao.org/publications/sofa/2009/en/ (accessed February 18, 2010).
Faverdin, P., Guyomard, H., Puillet, L., and Forslund, A. (2022). Animal board invited review: specialising and intensifying cattle production for better efficiency and less global warming: contrasting results for milk and meat co-production at different scales. Animal 16, 100431. doi: 10.1016/j.animal.2021.100431
Feder, G. (1985). The relation between farm size and farm productivity: the role of family labor, supervision and credit constraints. J. Dev. Econ. 18, 297–313. doi: 10.1016/0304-3878(85)90059-8
Ferreira, M. D. P., and Féres, J. G. (2020). Farm size and land use efficiency in the Brazilian Amazon. Land Use Policy 99, 104901. doi: 10.1016/j.landusepol.2020.104901
Gargiulo, J. I., Eastwood, C. R., Garcia, S. C., and Lyons, N. A. (2018). Dairy farmers with larger herd sizes adopt more precision dairy technologies. J. Dairy Sci. 101, 5466–5473. doi: 10.3168/jds.2017-13324
Gaude, I., Kempf, A., Strüve, K. D., and Hoedemaker, M. (2021). Estrus signs in Holstein Friesian dairy cows and their reliability for ovulation detection in the context of visual estrus detection. Livest. Sci. 245, 104449. doi: 10.1016/j.livsci.2021.104449
Guo, Q., Xue, J., and Gao, R. (2022). A comparative study of dual circulation development pattern in china and the united states. Am. J. Ind. Bus. Manag. 12, 1047–1066. doi: 10.4236/ajibm.2022.125055
Helfand, S. M., and Taylor, M. P. H. (2021). The inverse relationship between farm size and productivity: refocusing the debate. Food Policy 99, 101977. doi: 10.1016/j.foodpol.2020.101977
Herron, J., O'Brien, D., and Shalloo, L. (2022). Life cycle assessment of pasture-based dairy production systems: current and future performance. J. Dairy Sci. 105, 5849–5869. doi: 10.3168/jds.2021-21499
Homer, E. M., Gao, Y., Meng, X., Dodson, A., Webb, R., Garnsworthy, P. C., et al. (2013). Technical note: a novel approach to the detection of estrus in dairy cows using ultra-wideband technology. J. Dairy Sci. 96, 6529–6534. doi: 10.3168/jds.2013-6747
Hu, L., Zhang, X., and Zhou, Y. (2019). Farm size and fertilizer sustainable use: an empirical study in Jiangsu, China. J. Integr. Agr. 18, 2898–2909. doi: 10.1016/S2095-3119(19)62732-2
Huang, Y., Chen, Z., Yu, T., Huang, X., and Gu, X. (2018). Agricultural remote sensing big data: management and applications. J. Integr. Agr. 17, 1915–1931. doi: 10.1016/S2095-3119(17)61859-8
Huettel, S., and Jongeneel, R. (2011). How has the EU milk quota affected patterns of herd-size change? Eur. Rev. Agric. Econ. 38, 497–527. doi: 10.1093/erae/jbq050
Julien, J. C., Bravo-Ureta, B. E., and Rada, N. E. (2021). Productive efficiency and farm size in East Africa. Agrekon. 60, 209–226. doi: 10.1080/03031853.2021.1960176
Kato, H., Ono, H., Sato, M., Noguchi, M., and Kobayashi, K. (2022). Relationships between management factors in dairy production systems and mental health of farm managers in Japan. J. Dairy Sci. 105, 441–452. doi: 10.3168/jds.2021-20666
Kelly, P., Shalloo, L., Wallace, M., and Dillon, P. (2020). The Irish dairy industry - recent history and strategy, current state and future challenges. Int. J. Dairy Technol. 73, 309–323. doi: 10.1111/1471-0307.12682
Khanal, A. R., Gillespie, J., and Macdonald, J. (2010). Adoption of technology, management practices, and production systems in US milk production. J. Dairy Sci. 93, 6012–6022. doi: 10.3168/jds.2010-3425
Kolady, D. E., Van der Sluis, E., Uddin, M. M., and Deutz, A. P. (2021). Determinants of adoption and adoption intensity of precision agriculture technologies: evidence from South Dakota. Precis. Agric. 22, 689–710. doi: 10.1007/s11119-020-09750-2
Krpalkova, L., Cabrera, V. E., Kvapilik, J., and Burdych, J. (2016). Dairy farm profit according to the herd size, milk yield, and number of cows per worker. Agric. Econ. 62, 225–234. doi: 10.17221/126/2015-AGRICECON
Kumar, A., Mishra, A. K., Saroj, S., Sonkar, V. K., Thapa, G., Joshi, P. K., et al. (2020). Food safety measures and food security of smallholder dairy farmers: empirical evidence from Bihar, India. Agribusiness 36, 363–384. doi: 10.1002/agr.21643
Lagrange, V., Whitsett, D., and Burris, C. (2015). Global market for dairy proteins. J. Food Sci. 80, A16–22. doi: 10.1111/1750-3841.12801
Lambert, D. M., Paudel, K. P., and Larson, J. A. (2015). Bundled adoption of precision agriculture technologies by cotton producers. J. Agr. Resour. Econ. 40, 325–345. Available online at: https://www.jstor.org/stable/44131864
Lamkowsky, M., Oenema, O., Meuwissen, M. P. M., and Ang, F. (2021). Closing productivity gaps among Dutch dairy farms can boost profit and reduce nitrogen pollution. Environ. Res. Lett. 16, 124003. doi: 10.1088/1748-9326/ac3286
Läpple, D., Renwick, A., and Thorne, F. (2015). Measuring and understanding the drivers of agricultural innovation: evidence from Ireland. Food Policy 51, 1–8. doi: 10.1016/j.foodpol.2014.11.003
Lerman, Z. (2008). Agricultural development in central Asia: a survey of Uzbekistan, 2007-2008. Eurasian Geogr. Econ. 49, 481–505. doi: 10.2747/1539-7216.49.4.481
Li, X. (2017). Effect of System Vacuum of Milking Machine and Milk Flow Threshold of Automatic Cluster Remover on Udder Health [Dissertation/Doctoral Thesis]. Beijing: China Agricultural University (in Chinese).
Lin, J. Y., and Wang, X. (2021). Dual circulation: a new structural economics view of development. J. Chin. Econ. Bus. Stud. 20, 1–20. doi: 10.1080/14765284.2021.1929793
Liu, C., Han, L., and Zhang, Y. (2018). International comparison and development suggestions of China's dairy industry competitiveness. Chinese Rural Economy. 7, 130–144 (in Chinese). Available online at: https://kns.cnki.net/kcms/detail/11.1262.F.20180801.1001.018.html
Liu, Z., Zhai, T., and He, D. (2019). Research status and progress of individual information monitoring of dairy cows in precision breeding. Heilong Anim. Sci. Vet. Med. 13, 30–38. doi: 10.13881/j.cnki.hljxmsy.2019.01.0378 (in Chinese).
Luo, X., Wang, X., Shen, J., Qian, D., and Ye, F. (2019). The application of SCR estrus detection in the dairy reproduction system. J. Zhejiang Agric. Sci. 60, 2380–2381 (in Chinese). doi: 10.16178/j.issn.0528-9017.20191261
Lyytinen, K., Yoo, Y., and Boland Jr, R. J. (2016). Digital product innovation within four classes of innovation networks. Inf. Syst. J. 26, 47–75. doi: 10.1111/isj.12093
Ma, M., Lin, J., and Sexton, R. J. (2022). The transition from small to large farms in developing economies: a welfare analysis. Am. J. Agr. Econ. 104, 111–133. doi: 10.1111/ajae.12195
Ma, W., Renwick, A., and Greig, B. (2019). Modelling the heterogeneous effects of stocking rate on dairy production: an application of unconditional quantile regression with fixed effects. Appl Econom. 51, 4769–4780. doi: 10.1080/00036846.2019.1602710
Mao, H., Zhou, L., Ying, R., and Pan, D. (2021). Time preferences and green agricultural technology adoption: field evidence from rice farmers in China. Land Use Policy. 109, 105627. doi: 10.1016/j.landusepol.2021.105627
Mayo, L. M., Silvia, W. J., Ray, D. L., Jones, B. W., Stone, A. E., Tsai, I. C., et al. (2019). Automated estrous detection using multiple commercial precision dairy monitoring technologies in synchronized dairy cows. J. Dairy Sci. 102, 2645–2656. doi: 10.3168/jds.2018-14738
MEWEC (Market Early Warning Expert Committee Ministry of Agriculture and Rural Affairs).. (2021). China Agricultural Outlook 2021-2030. Beijing: China Agricultural Science and Technology Press.
Min, S., Liu, M., and Huang, J. (2020). Does the application of ICTs facilitate rural economic transformation in China? Empirical evidence from the use of smartphones among farmers. J. Asian Econ. 70, 101219. doi: 10.1016/j.asieco.2020.101219
Mo, D., Huang, J., Jia, X., Luan, H., Rozelle, S., Swinnen, J., et al. (2012). Checking into China's cow hotels: have policies following the milk scandal changed the structure of the dairy sector? J. Dairy Sci. 95, 2282–2298. doi: 10.3168/jds.2011-4720
Norring, M., Valros, A., and Munksgaard, L. (2012). Milk yield affects time budget of dairy cows in tie-stalls. J. Dairy Sci. 95, 102–108. doi: 10.3168/jds.2010-3458
Otsuka, K., Liu, Y., and Yamauchi, F. (2016). Growing advantage of large farms in Asia and its implications for global food security. Glob. Food Secur. 11, 5–10. doi: 10.1016/j.gfs.2016.03.001
Parikoglou, I., Emvalomatis, G., and Thorne, F. (2022). Precision livestock agriculture and productive efficiency: the case of milk recording in Ireland. Agr. Econ. 1–12. doi: 10.1111/agec.12729
PDNDRC (Price Department of National Development and Reform Commission). (2021). National Compilation of Agricultural Product Costs and Benefits 2021. Beijing: China Statistics Press.
Peng, H., and Li, J. (2020). The application of mechanization, intelligence and informatization in dairy farming in China. Food Nutr. China 26, 5–9. doi: 10.19870/j.cnki.11-3716/ts.20200909.001
Pierpaoli, E., Carli, G., Pignatti, E., and Canavari, M. (2013). Drivers of precision agriculture technologies adoption: a literature review. Procedia Technol. 8, 61–69. doi: 10.1016/j.protcy.2013.11.010
Pivoto, D., Barham, B., Waquil, P. D., Foguesatto, C. R., Corte, V. F. D., Zhang, D., et al. (2019). Factors influencing the adoption of smart farming by Brazilian grain farmers. Int. Food Agribus. Man. 22, 571–588. doi: 10.22434/IFAMR2018.0086
Rada, N. E., and Fuglie, K. O. (2019). New perspectives on farm size and productivity. Food Policy 84, 147–152. doi: 10.1016/j.foodpol.2018.03.015
Reith, S., and Hoy, S. (2018). Review: behavioral signs of estrus and the potential of fully automated systems for detection of estrus in dairy cattle. Animal 12, 398–407. doi: 10.1017/S1751731117001975
Roelofs, J., López-Gatius, F., Hunter, R. H. F., van Eerdenburg, F. J. C. M., and Hanzen, C. (2010). When is a cow in estrus? Clinical and practical aspects. Theriogenology 74, 327–344. doi: 10.1016/j.theriogenology.2010.02.016
Rorie, R. W., Bilby, T. R., and Lester, T. D. (2002). Application of electronic estrus detection technologies to reproductive management of cattle. Theriogenology 57, 137–148. doi: 10.1016/S0093-691X(01)00663-X
Sen, A. (1962). An aspect of Indian agriculture. Economic Weekly. 14, 243–246. Available online at: https://www.epw.in/system/files/pdf/1962_14/4-5-6/an_aspect_of_indian_agriculture.pdf
Shen, Z., Wang, S., Boussemart, J., and Hao, Y. (2022). Digital transition and green growth in Chinese agriculture. Technol. Forecast. Soc. Change 181, 121742. doi: 10.1016/j.techfore.2022.121742
Sheng, Y., Ding, J., and Huang, J. (2018). The Relationship between farm size and productivity in agriculture: evidence from Maize Production in Northern China. Am. J. Agr. Econ. 101, 790–806. doi: 10.1093/ajae/aay104
Smith, M. J. (2020). Getting value from artificial intelligence in agriculture. Anim. Prod. Sci. 60, 46–54. doi: 10.1071/AN18522
Speicher, J. A., Nott, S. B., and Stoll, T. L. (1978). Changes in production, cash flow, and income with dairy herd expansion. J. Dairy Sci. 61, 1242–1249. doi: 10.3168/jds.S0022-0302(78)83713-8
Steeneveld, W., Hogeveen, H., and Oude Lansink, A. G. J. M. (2015). Economic consequences of investing in sensor systems on dairy farms. Comput. Electron. Agr. 119, 33–39. doi: 10.1016/j.compag.2015.10.006
Stevenson, J. S., Hill, S. L., Nebel, R. L., and DeJarnette, J. M. (2014). Ovulation timing and conception risk after automated activity monitoring in lactating dairy cows. J. Dairy Sci. 97, 4296–4308. doi: 10.3168/jds.2013-7873
Stock, J., and Yogo, M. (2005). Testing for Weak Instruments in Linear IV Regression. Cambridge: Cambridge University Press. doi: 10.1017/CBO9780511614491.006
Tamirat, T. W., Pedersen, S. M., and Lind, K. M. (2018). Farm and operator characteristics affecting adoption of precision agriculture in Denmark and Germany. Acta Agric. Scand. B Soil Plant Sci. 68, 349–357. doi: 10.1080/09064710.2017.1402949
von Briel, F., Davidsson, P., and Recker, J. (2018). Digital technologies as external enablers of new venture creation in the IT hardware sector. Entrep. Theory Pract. 42, 47–69. doi: 10.1177/1042258717732779
Wang, X., Yamauchi, F., and Huang, J. (2016). Rising wages, mechanization, and the substitution between capital and labor: evidence from small scale farm system in China. Agr. Econ. 47, 309–317. doi: 10.1111/agec.12231
Weersink, A., and Tauer, L. W. (1991). Causality between dairy farm size and productivity. Am. J. Agric. Econ. 73, 1138–1145. doi: 10.2307/1242442
Werkheiser, I. (2018). Precision livestock farming and farmers' duties to livestock. J. Agric. Environ. Ethics 31, 181–195. doi: 10.1007/s10806-018-9720-0
Xia, F., Hou, L., Jin, S., and Li, D. (2020). Land size and productivity in the livestock sector: evidence from pastoral areas in China. Aust. J. Agr. Resour. Econ. 64, 867–888. doi: 10.1111/1467-8489.12381
Xie, L., Luo, B., and Zhong, W. (2021). How are smallholder farmers involved in digital agriculture in developing countries: a case study from China. Land 10, 245. doi: 10.3390/land10030245
Yamauchi, F. (2016). Rising real wages, mechanization and growing advantage of large farms: evidence from Indonesia. Food Policy 58, 62–69. doi: 10.1016/j.foodpol.2015.11.004
Yang, W., Edwards, J. P., Eastwood, C. R., Dela Rue, B. T., and Renwick, A. (2021). Analysis of adoption trends of in-parlor technologies over a 10-year period for labor saving and data capture on pasture-based dairy farms. J. Dairy Sci. 104, 431–442. doi: 10.3168/jds.2020-18726
Yu, X. (2012). Productivity, efficiency and structural problems in Chinese dairy farms. China Agr. Econ. Rev. 4, 168–175. doi: 10.1108/17561371211224755
Keywords: herd size, milk production, productivity, digital technology, large-scale farms, sustainable foods production
Citation: Qi Y, Han J, Shadbolt NM and Zhang Q (2022) Can the use of digital technology improve the cow milk productivity in large dairy herds? Evidence from China's Shandong Province. Front. Sustain. Food Syst. 6:1083906. doi: 10.3389/fsufs.2022.1083906
Received: 29 October 2022; Accepted: 16 November 2022;
Published: 02 December 2022.
Edited by:
Muhammad Asad Ur Rehman Naseer, Bahauddin Zakariya University, PakistanReviewed by:
Xiaoheng Zhang, Nanjing University of Aeronautics and Astronautics, ChinaHui Mao, Shaanxi Normal University, China
Copyright © 2022 Qi, Han, Shadbolt and Zhang. This is an open-access article distributed under the terms of the Creative Commons Attribution License (CC BY). The use, distribution or reproduction in other forums is permitted, provided the original author(s) and the copyright owner(s) are credited and that the original publication in this journal is cited, in accordance with accepted academic practice. No use, distribution or reproduction is permitted which does not comply with these terms.
*Correspondence: Jiqin Han, jhan@njau.edu.cn; Qing Zhang, zhangqing1983@webmail.hzau.edu.cn